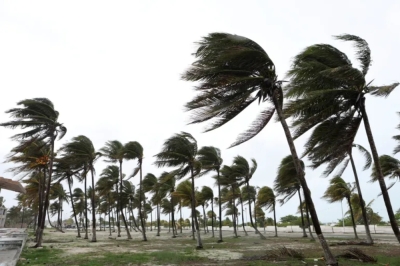
The Problem of Automated Screening of Job Applicants
There are plenty of good things that happen when firms post their job openings online.
When a job is posted publicly, many more people have a chance to learn about it. Jobs are perhaps less likely to be allocated by a social network of who-hears-what-from-whom, opening up opportunities more broadly. Also, potential workers can search jobs and employers with their computer or phone, rather than needing to travel in-person from one potential employer to another, gathering up applications. Filling out applications on-line can be quicker than filling out paper forms in person, too.
But there’s also a long-standing problem with online job applications, which in an essay written 20 years ago David Autor called the problem of “excess application.” That is, people can easily apply for many more jobs, and as a result, employers get many more applications for a given job. Employers need to whittle down the online job applicants to a manageable number, so they turn to automated tools for screening the job applications. Joseph B. Fuller, Manjari Raman, Eva Sage-Gavin, and Kristen Hines describe some of the problems that arise in “Hidden Workers: Untapped Talent,” subtitled “How leaders can improve hiring practices to uncover missed talent pools, close skills gaps, and improve diversity (published by Accenture and the Harvard Business School, September 2021). They write:
An Applicant Tracking System (ATS) is a workflow-oriented tool that helps organizations manage and track the pipeline of applicants in each step of the recruiting process. A Recruiting Management or Marketing System (RMS) complements the ATS and supports recruiters in all activities related to marketing open positions, sourcing key talent,
creating talent pools, and automating aspects of the recruiting process such as automates candidate scoring and interview scheduling. Together, these systems represent the foundation of the hiring process in a majority of organizations. In fact, more than 90% of employers in our survey use their RMS to initially filter or rank potential middle-skills (94%) and high-skills (92%) candidates. These systems are vital; however, they are designed to maximize the efficiency of the process. That leads them to hone in on candidates, using very specific parameters, in order to minimize the number of applicants that are actively considered. For example, most use proxies (such as a college degree or possession of precisely described skills) for attributes such as skills, work ethic, and self-efficacy. Most also use a failure to meet certain criteria (such as a gap in full-time employment) as a basis for excluding a candidate from consideration irrespective of their other qualifications.
As a result, they exclude from consideration viable candidates whose resumes do not match the criteria but who could perform at a high level with training. A large majority (88%) of employers agree, telling us that qualified high skills candidates are vetted out of the process because they do not match the exact criteria established by the job description. That number rose to 94% in the case of middle-skills workers.
Thus, the problem of excess applications requires that firms do automated screening. But all too often, the automated screening relies on using certain keywords, which are only a rough proxy for what the firm is looking for. The report has several suggestions for addressing this problem: for example, firms should avoid job descriptions with a long list of “nice-to-haves” that end up ruling out lots of potential workers, and focus on a shorter list of “must-haves.” But remember, the beginning of the problem is that the firm needs ways of reducing the excess applications to a manageable number, and having fewer job requirements doesn’t address that need. Thus, actual tests of the must-have skills can be a useful way of whittling down the list of applicants
Some human resources expert argue that human resources departments in general often do a poor job in the interview process as well. Interviewers tend to like people who are like themselves. Some of the metrics often used for hiring young adults, like college grades, don’t have a strong correlation with workplace performance. Many companies don’t have a process for looking at basic data about recently hired employees–stuff like absence and quit rates, performance-based raises, or just asking the supervisor if they would hire that person again. With that information a firm could then work backward to see if some common traits could have been helpful in the hiring process, and perhaps also what “nice-to-have” traits didn’t turn out to be all that important.
In short, the goal is to have firms rethink the automated screening processes they are using, and come up with alternative ways of shaping the applicant pool–broadening it in some ways, but also narrowing it in different ways–so that the result is more likely to lead to a productive and lasting job match. The Accenture/HBS report argues that companies that have developed ways to reach out to the “hidden workers” who often will not make it through current automated screening of job applications “were 36% less likely to
face talent and skills shortages compared to companies that do not hire hidden workers.”
Following this line of thought, I was intrigued by “Hiring as Exploration,” by Danielle Li, Lindsey R. Raymond, and Peter Bergman (NBER Working Paper 27736, August 2020). They consider a “contextual bandit” approach. The intuition here, at least as I learned it, refers to the idea of a “one-armed bandit” as a synonym for a slot machine. Say that you are confronted with the problem of which slot machine to play in a large casino, given that some slot machines will pay off better than others. On one side, you want to exploit a slot machine with high payoffs. On the other hand, even if you find a slot machine which seems to have pretty good payoffs, it can be a useful strategy to explore a little and see if perhaps some unexpected slot machine might pay as well or better. A contextual bandit model is built on finding the appropriate balance in this exploit/explore dynamic.
From this perspective, the problem with a lot of automated methods for screening job applications is that they do too little exploring. The automated tools take whatever criteria are imposed and narrow down this list of candidates, but they don’t systematically sample those who are perhaps fairly close to being on the final list, but being left off, to learn about other mixtures of personal backgrounds, skills, and traits that might be important.
In this spirit, the authors create several algorithms for screening job applicants, and they define an applicant’s “hiring potential” as the likelihood that the person will be hired, given that they are interviewed. The algorithms all use background information “on an applicant’s demographics (race, gender, and ethnicity), education (institution and degree), and work history (prior films).” The key difference is that some of the algorithms just produce a point score for who should be interviewed, while the contextual bandit algorithm produces both a point score as a standard deviation around that point score. Then, and here is the key point, the contextual bandit algorithm ranks the applicants according to the upper bound of the confidence interval associated with the standard deviation. Thus, an applicant with a lower score but higher uncertainty could easily be ranked ahead of an applicant with a higher score but lower uncertainty. Again, the idea is to get more exploration into the job search and to look for matches that might be exceptionally good, even at the risk of interviewing some real duds. They apply their algorithms to actual job applicants for professional services jobs at a Fortune 500 firm. They write:
Our data come from administrative records on job applications to these types of professional services positions within a Fortune 500 firm. Like many other firms in its sector, this firm is overwhelmed with applications and rejects the vast majority of candidates on the basis of an initial resume screen. Yet, among those who pass this screen and go on to be interviewed, hiring rates are still relatively low: in our case, only 10% receive and accept an offer. Because recruiting is costly and diverts employees from other productive work, the firm would like to adopt screening tools that improve its ability to identify applicants it may actually hire.”
They find that several of the algorithms would have the effect of reducing the share of selected applicants who are Black or Hispanic, while the contextual bandit approach looking for employees who are potentially outstanding “would more than double the share of selected applicants who are Black or Hispanic, from 10% to 23%.” They also find that while the previous approach at this firm was leading to a situation where 10% of those interviewed were actually offered and accepted a job, the contextual bandit approach led to an applicant pool where 25% of those who were interviewed were offered and accepted a job.
In short, automated screening of job applications is a practical necessity, but too many firms are using it in a way that limits their applicant pool in undesired ways–and then those same firms are more likely to complain that they don’t have enough qualified applicants. For those firms, perhaps the problem doesn’t rest with the pool of job applicants, but in the unthoughtful and counterproductive ways the potential employers are fishing in that pool.
Trending
-
1 UK Tech Sector Secures a Third of European VC Funding in 2024
Azamat Abdoullaev -
2 France’s Main Problem is Socialism, Not Elections
Daniel Lacalle -
3 Fed Chair Jerome Powell Reports 'Modest' Progress in Inflation Fight
Daniel Lacalle -
4 AI Investments Drive 47% Increase in US Venture Capital Funding
Felix Yim -
5 The Future of Work: How Significance Drives Employee Engagement
Daniel Burrus
Comments