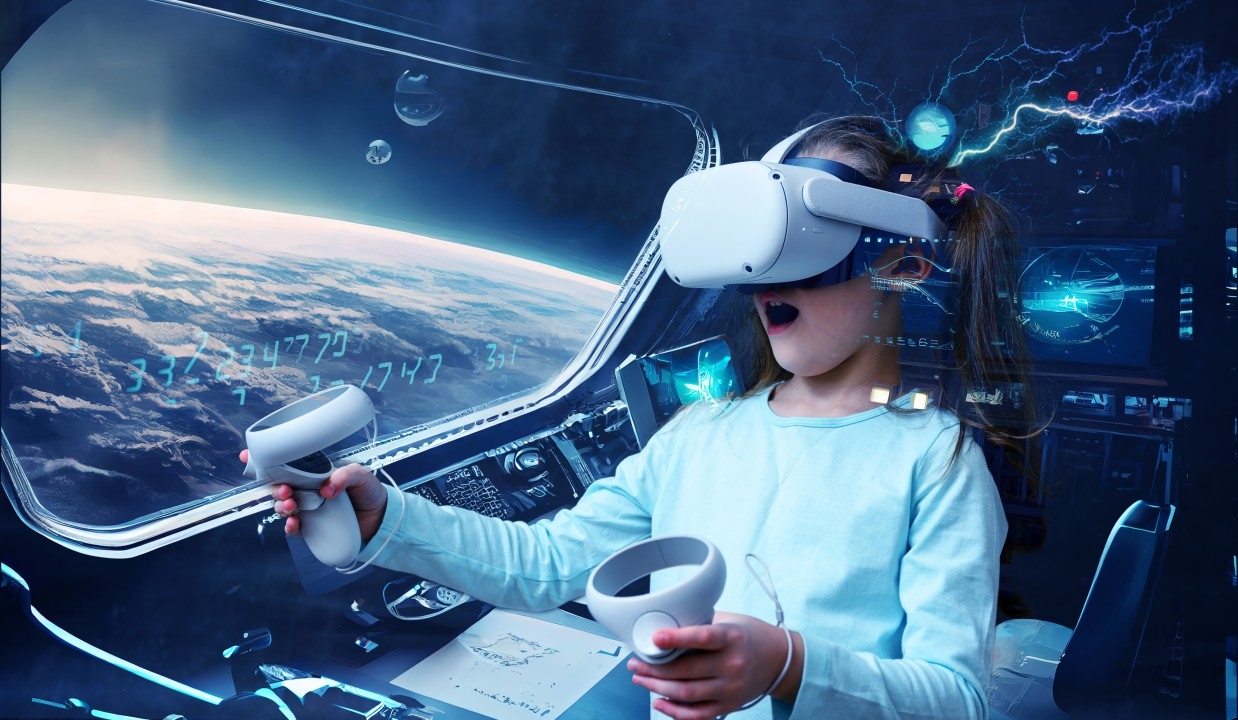
Evolution of Artificial Intelligence in Marketing and Sales
According to Teradata’s ‘State of AI for Enterprises’ report in October 2017, 80% of enterprises have active AI in production today, with Asia-Pacific leading all regions. With all the major tech companies reorienting themselves around AI and the announcement of self-driven cars from several automobile majors, we can say with conviction, that the AI wave has arrived.
With its Azure platform, Microsoft is taking a big step forward in helping businesses harness this technology. As Microsoft mentioned at the Ignite event, its goal is to support businesses in the areas of customer service and sales support. Through its Dynamics 365 AI solution, which is being tested with companies such as HP and Macy’s, AI is being used to help customers get the products and services they need.
Impact of AI on Marketing and Sales
The evolution of AI is evident because, lately the discussions in the data science space, is not just filled with data modeling issues, but also with building new machine learning-specific platforms with end-to-end functionality.
With the need for greater efficiency every day; marketing and sales are at the center of the AI evolution. Companies that vastly rely on inbound leads and inbound marketing practices, rely on customer data to understand customer behavior. The importance of understanding the customer journey, and the need to improve customer experience continuously, are things that don’t need emphasis any more. Similarly, outbound teams are also using AI to a significant advantage in streamlining their CRM systems, thus ensuring that their sales teams are focusing on the right leads that have the highest sales propensity.
From the machine learning perspective, it all starts with data processing, followed by the subsequent stages model building and deployment & monitoring.
Data Processing Stage
The data processing stage typically involves collecting, cleaning and formatting data which can be fed into the models. Models are as good as the data inputs, which makes the data processing supremely critical for marketers and salespeople. At this stage, most companies use a combination SQL queries and Python scripts to aggregate and format data from multiple sources.
Data Modeling, Deployment, and Monitoring
The data modeling process involves understanding and paying attention to what is important in the data, to answer the questions asked, and find a model that fits the best to make predictions. The challenge here is that the real-world data may decrease the accuracy of trained models, which means data scientists have to re-train production models on fresh data on a daily to monthly basis, depending on the application.
When it comes to deployment, engineers tend to save a serialized version of the trained model or model weights to a file. Engineers sometimes need to rebuild model prototypes and parts of the data pipeline in a different language or framework, so they work on production infrastructure.
Given that any incompatibility issue at any stage can introduce error, several companies are investing in building their own custom tools that work with their infrastructure. There are services like Azure Machine Learning and Amazon Machine Learning that are publicly available alternatives, but they integrate with other Amazon or Microsoft services for the data storage and deployment components. It means they are a good fit only if your infrastructure is in Amazon or Microsoft stack.
The Real Issue and the Way Forward
For marketers and salespeople, the actual pain point is getting the right information about the prospect or customer at the right time, and intelligence & insight into what interests them and why. For example, as a salesperson, you might want to know which are the accounts that are likely to convert into sales opportunities. Similarly, a marketer might be interested in knowing what content will likely resonate the most, for the prospect.
This means AI needs to be able to monitor customer behavior, understand their intent, know if the product offering is a good fit and thus offer a 360° customer insight. More importantly, the results generated have to be dynamic and adaptable to the current changes at the prospect’s company.
Fortunately, with the current focus on AI across the spectrum, companies are investing on specialized tools such as Fiind Smart Signals platform, that enable marketing and sales people to find their next customer. Tools such as these integrate tightly into their existing infrastructure so that there is no need to boil the ocean to get the right results at scale.
AI Adoption for Marketing and Sales Will be More than Ever in 2019
For marketers and more so for growth hackers, AI is going to be a huge enabler. However, given that every organization is different and their needs are different, point solutions will have to co-exist. We might likely move to a space where specialized AI tools integrate with other AI vendors as well, to offer a consolidated data view across your enterprise.
Double your investments on AI, it is here to stay!
Comments