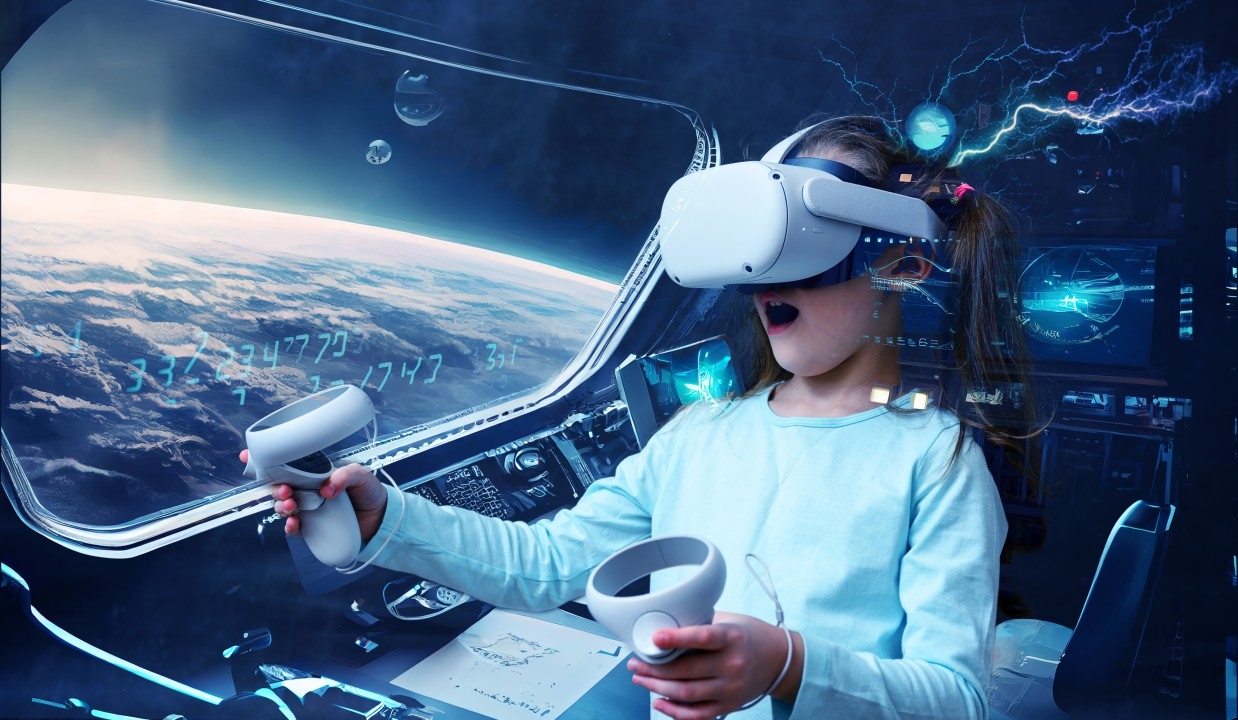
Generative AI: How LLMs Enhance Customer Experience & Business Value
The rise of the LLM and Generative AI models brings the era of mass hyper personalisation at scale.
Increasingly customers are expecting fast and effective responses whether in relation to customer support matters or for helpful and tailored recommendations, product search and discovery and information.
Large Language Models (LLMs) have been key for advancing Generative AI as the natural language capabilities of computing machines has rapidly advanced.
Advancing computing capabilities across language has been a key aspect of AI development in recent times and is set to continue in 2024. Language capabilities for AI agents brings the ability to personalise the engagement for the customer and within the internal operation side for the enterprise staff to assist them in their work and expedite tasks more efficiently.
McKinsey have estimated in a report entitled ‘The economic potential of generative AI: The next productivity frontier’ that Generative AI may add up to $2.6 to $4.4 trillion to the global economy on an annual basis and that potentially up to ¾ of the total annual value of use cases will result marketing and sales, customer operations, R&D and software engineering.
BCG points out how Generative AI may generate content, improve efficiency (For example summarise documents) and personalise experiences by identifying patterns in a given customer’s behaviour and generating tailored information for that particular person. However, BCG also notes that the extended adoption of Generative AI may result in challenges for organisations that have not implemented suitable governance arrangements. These include bias and toxicity, data leakage whereby sensitive, proprietary data may be entered into a Generative AI model and reappear in the public domain, and a lack of transparency.
Therefore, it is important for businesses that their offerings provide reliable and trustworthy solutions for their external customers and internal operational needs. With this in mind it is worth considering OTTES:
-
Open: whereby the model has enough variation to meet the needs of enterprise use cases and requirements for compliance obligations.
-
Trusted: whereby customers may apply their own proprietary data, develop an AI model and, in the process, ensure that ethical, regulatory and legal matters are managed appropriately.
-
Targeted: solution that is designed for business use cases with the intention to unlock new value.
-
Empowering: the model allows the user to become a value creator across training, fine-tuning, deployment and data governance.
-
Sustainability: working with a LLM provider who is committed to achieving net-zero emissions of greenhouse gasses.
I am delighted to collaborate with IBM watsonx and to set out how OTTE with an LLM is enabled by watsonx. Furthermore, I am also delighted that IBM have committed to sustainability with a target to hit net-zero operational greenhouse gasses by 2030 and have reported that the firm reduced operational greenhouse gas emissions by 61% since 2010;
In addition, IBM have set out the following core principles for Generative AI for enterprise:
What is Natural Language Processing (NLP)? And what is a Large Language Model (LLM)
NLP is the area of computer science and in particular Artificial Intelligence (AI) that enables computers the ability to understand text and speech in a similar manner to humans.
Natural Language Understanding (NLU) and Natural Language Generation (NLG) are subsets of NLP. NLU focuses on deriving understanding from language (speech or text). NLG is applied towards the generation of language (speech or text) that is capable of being understood by humans.
LLM refers to a type of foundation model that has been trained on a vast set of data enabling the model to understand and generate NLP related tasks and even wider tasks (multimodal, multitasking).
Many firms, including IBM, have been developing NLP capabilities over an extended period of time along with the powerful Machine Learning and in particular the Deep Neural Network architecture required for advancing NLP. In particular the Transformer with Self-Attention Mechanism that LLMs typically apply.
The paper Attention is All you need (2017) introduced the concept and for more on how Transformers with the Self-Attention Mechanism work see:
-
The Transformer Attention Mechanism (for a more technical overview).
Transformers are giant models and building a foundation LLM model from scratch requires large amounts of data and powerful server capabilities, and software engineering expertise (data engineers, natural language processing experts).
The attention of the public and many C-Teams has been captured by the likes of Open AI’s Chat GPT-3 and GPT-4, and the developer community has also been excited by the likes of Meta’s Llama models and other models available via open source.
There are firms who seek easily implementable solutions from a reliable and trustworthy enterprise supplier with a strong balance sheet.
Furthermore, there are those firms from the Small and Medium Enterprise (SME) segments who could massively benefit from the opportunities that LLMs offer but don’t have the internal resources to access and scale the more complex models.
For firms in the above categories seeking a solution from a reliable and secure counterparty, IBM have launched the Granite model series on watsonx.ai as the Generative AI offering from IBM services including watsonx Assistant and watsonx Orchestrate.
Application of LLMs
LLMs provide understanding and text generation as well as further types of content generation utilising huge amounts of data for the purposes of training. From a use case perspective, LLMs may infer from context, coherently generate responses that are relevant to the context as well as text summarization, language translation, sentiment analysis, Q&A answering, code generation as well as writing creatively.
This ability is due to the vast size of the LLMs (often billions of parameters) and building and training an LLM from scratch is typically a task that will require significant resources (data, time, engineers, servers with GPUs and money). Hence the reason why firms (in particular those that are outside of the tech sector) are using services from LLMs available in the market.
The section below sets out how, according to IBM, watsonx may enable the user to work with LLM technology that is secure, reliable and relatively easy to adopt.
An Overview of Watsonx
-
watsonx.ai: maybe considered as a studio for foundation models and generative AI capabilities. According to IBM it allows the user to train, validate, tune and deploy foundation models for Generative AI purposes as well as Machine Learning tools with a material saving in time and data
-
watsonx.data: applies open lakehouse architecture to provide a data store. This architecture is optimized for AI workloads as well as governed data with users given access enabling them to query and access open data formats in addition to engaging governance. Furthermore, watsonx.data enables the user to manage workloads across multi cloud environments as well as on-premises with an estimate from IBM that users may be able to reduce their warehouse costs by up to 50% by using watsonx.data. A further advantage is that it gives tools that are built in for integrations, automation and governance.
-
A further point about watsonx.data according to IBM is that it provides for an integrated vectorized embedding capability to prepare the users’ data for Retrieval Augment Generation (RAG) or other machine learning and generative AI use cases (in tech preview).
-
RAG is increasingly important in enabling LLM models to return the relevant response in natural language by searching enterprise documents that are up to date. According to IBM the user may apply foundation models in watsonx.ai to generate factually accurate output that is grounded in information in a knowledge base by applying the RAG pattern.
-
See the following link for a step by step overview of RAG in a notebook with watson.x.
-
watsonx.governance: gives tool for responsible AI workflows. According to IBM this service will enable protection of customer privacy as well as detection of drift and bias within models as well as assisting firms to maintain their standards of ethics. Moreover, according to IBM the advantages for the user include a reduction in time, cost and risk entailed in manual processes with documentation resulting in explainable outcomes and transparency.
-
Moreover, an example of ethical AI implementation is provided by Dun & Bradstreet and IBM: Pioneering Ethical AI in Business Insights Powered by watsonx.
Further advantages of IBM watsonx are set out below:
-
IBM have a track record in funding ongoing research into advancing AI capabilities for example Paul Smith Goodson observed that “IBM has one of the largest and most well-funded AI research programs in the world” in IBM Demonstrates Groundbreaking Artificial Intelligence Research Using Foundational Models And Generative AI.
-
IBM watsonx is differentiated from the likes of the Open AI Chat GPT model in the sense that it was primarily intended for the enterprise market and hence watsonx enables its users to fine-tune, train, deploy and crucially govern the data and AI models brought to the platform by the enterprise. In this sense the enterprise fully owns the value creation part.
-
watsonx Assistant provides a summary of the interactions between users and the virtual agent deployed by the enterprise. Visualization and analysis of critical metrics and KPIs empower the firm to understand what users and customers want addressed, whether those needs are being met by the virtual agent, and how to rapidly improve the service provided by the virtual agent.
-
Furthermore, the watsonx Assistant, enables subject matter experts to build and maintain advanced conversational flows without the need for programming knowledge as they may simply drag and drop. This is further enabled for those in the banking sector with banking-specific templates and documentation for getting started, integrations, and dialog flow get them up and running quickly.
-
In addition, watsonx provides aSynthetic Data Generator to generate synthetic tabular data based on production data or a custom data schema using visual flows and modelling algorithms. Synthetic data can be applied towards mitigating ethical risks such as bias and governance issues such as data privacy.
In essence one may surmise that the key advantage of the Granite model series for enterprise is business-targeted, IBM-developed foundation models built from sound data.
Embeddable AI Partnership: Independent Software Company (ISV) and Managed Service Providers (MSP)
Furthermore, a software company can look at embedding watsonx into their AI commercial software solutions. Partnership opportunities allow for solutions to be elevated via hybrid cloud and AI technology allowing for expansion into new markets and established go-to market tools enabling strategies for sales amplification.
Moreover, the NLP solutions from watsonx may accelerate business value generated via AI through a flexible portfolio of services, applications and libraries. A particular advantage of the solution is that it makes the technology accessible to those who are not data scientists including non-technical users thereby enhancing productivity and business processes.
Use Case Examples: Retail, E-Commerce, Marketing, Advertising Sectors
Brands and firms can now engage with customers in a more meaningful and customised manner. LLM models can help provide useful information and helpful advice to potential customers about the product or service. Brands can use an LLM to create personalised campaigns and targeted promotions.
Furthermore, LLM models can be used to generate content whether social media posts and blogs or more creative idea content such as poetry that may engage the potential customer. Moreover, LLMs can be applied towards more capable and effective chatbots that can operate 24/7 and assist with customer queries thereby improving the customer experience and user engagement.
An example of enhanced customer support for the retail sector from watsonx-assistant is provided below, whereby the enterprise may inform every interaction, for example enable a customer to discover where the nearest stores are to them:
Source: IBM
A further example is to advise on every possibility: with a meaningful and useful proactive engagement to inform customers with recommendations and accurately inform them about the relevant possibilities.
Source: IBM
And to guide every experience: by accurately responding to customer inquiries that results in personalised guidance for the customer and captures sales leads in a meaningful manner by understanding customer intent and personalised follow-up engagement by an agent.
Source: IBM
Case studies are provided by:
-
Camping World implementation to reshape call centres that resulted in an increase in customer engagement by 40% across all platforms and reduced waiting times to 33 seconds with a 33% increase in agent efficiency reported.
-
Stiky providing 24x7 customer service, answering up to 90% of queries automatically, conducting on average 165conversations daily and 92% positive customer satisfaction rating (CSAT).
-
Bestseller India to boost fashion forecasting.
Furthermore, it is worth noting that according to Google “Consumers are 40% more likely to spend more than they originally planned when their retail experience is highly personalized to their needs.”
Use Case Examples: Banking Financial Services / Fintech
LLMs may provide insights from analyst reports, news articles, social media content and industry publications.
The watsonx service may be applied towards empowering customer self-service so that customers may gain rapid access to core banking actions such as searching branch locations, checking balances in their account, payments, transfers and independently resolve their support issues.
Source: IBM
A further example is provided by contextualizing experiences to drive outcomes in the banking experience for example providing suggestions that are relevant and guidance that is considered helpful as responses to the customer thereby improving the customer experience (CX).
Source: IBM
Moreover, the watsonx agent may also be applied towards suggesting helpful next steps whereby customers may be provided with intelligent recommendations and proactively informed about opportunities enabling them to accurately understand all the contextual possibilities.
Source: IBM
An advantage of this process is the delivery of human-like interactions irrespective of where the customer inquiry arrives, or the language spoken, with the examples provided by watsonx Assistant applying natural language processing (NLP) to enhance customer engagements towards those expected from human levels and quick responses (effectively in real-time).
Case study examples are provided by:
-
Citi transforming critical internal audit processes.
-
Just ask Anna virtual agent at ABNAmro.
-
GM Financial to develop a secure and powerful AI assistant.
-
Bradesco bank giving personal attention to its 65m customers with responses in seconds not minutes
-
Credit Mutuel providing expert service 60% faster
Use Case Examples: Insurance / Insurtech
Use case examples include agent assist that enables customers to ask basic queries such as the ability to reset password or seek information about their insurance policy and furthermore provide rapid responses to requests for quotes and pricing, coverage checks, processing of claims and the handling of matters relating to the policy.
Source: IBM
Moreover, insurance firms may also apply watsonx towards digital customer support to enable customer engagement and integrate an AI agent within existing systems and processes.
Source: IBM
Case study examples include:
-
Allianz creating an AI-powered virtual assistant that resulted in a high containment rate with a resolution of 80% of issues most frequently presented by customers to call centres and a reduced resolution time with common customer service requests being resolved within one to two minutes.
-
Across the industry: Cloud and AI make personalized insurance possible
-
Emerging risk concierges see almost half a billion USD more in annual revenue
IBM commissioned Forrester TEI and found that watsonx Assistant customers saw the following benefits:
-
$23 million in benefits over three years
-
Three-year 370% ROI
-
Cost savings of $6.00 per contained customer conversation
Increasingly enterprises are facing a race to adopt Generative AI capabilities or get left behind by their competitors, in particular if rivals can offer enhanced CX and customer engagement.
However, a business whether a large corporation or an SME must carefully evaluate which option and criteria best suits their needs. As noted, many firms will lack the internal resources and core competences to match a technology major to develop a state of the art LLM. Equally many such firms will seek to evaluate the open-source model solutions available with an evaluation of the architecture needs of a pipeline and engineering resources needed for a real-world production level system and governance requirements. For these firms a trusted counterparty who may deliver reliably and securely is key.
IBM watsonx have summarised how they meet OTTES criteria set out at the outset of this article:
-
Open: according to IBM, watsonx is based on the best open technologies available, providing model variety to cover enterprise use cases and compliance requirements.
-
Trusted: IBM state that watsonx is designed with principles of transparency, responsibility and governance enabling customers to build AI models trained on their own trusted data to help manage legal, regulatory, ethical and inaccuracy concerns.
-
Targeted: IBM point out that watsonx is designed for enterprise and targeted at business domains; watsonx is designed for business use cases that unlock new value.
-
Empowering: watsonx allows the user go beyond just being an AI user and become an AI value creator, allowing you to train, fine-tune and deploy, and govern the data and AI models you bring to the platform and own completely the value they create according to IBM.
-
Sustainability: as noted above, IBM targets to hit net-zero operational GHGs by 2030.
About the Author
Imtiaz Adam is a Hybrid Strategist and Data Scientist. He is focussed on the latest developments in artificial intelligence and machine learning techniques with a particular focus on deep learning. Imtiaz holds an MSc in Computer Science with research in AI (Distinction) University of London, MBA (Distinction), Sloan in Strategy Fellow London Business School, MSc Finance with Quantitative Econometric Modelling (Distinction) at Cass Business School. He is the Founder of Deep Learn Strategies Limited, and served as Director & Global Head of a business he founded at Morgan Stanley in Climate Finance & ESG Strategic Advisory. He has a strong expertise in enterprise sales & marketing, data science, and corporate & business strategist.
#IBMPartner
Source for performance data is IBM. Results may vary and are provided on a non-reliance basis and although the author has made reasonable efforts to research the information, the author makes no representations, warranties or guarantees, whether express or implied is provided in relation to the content in this article.
Comments