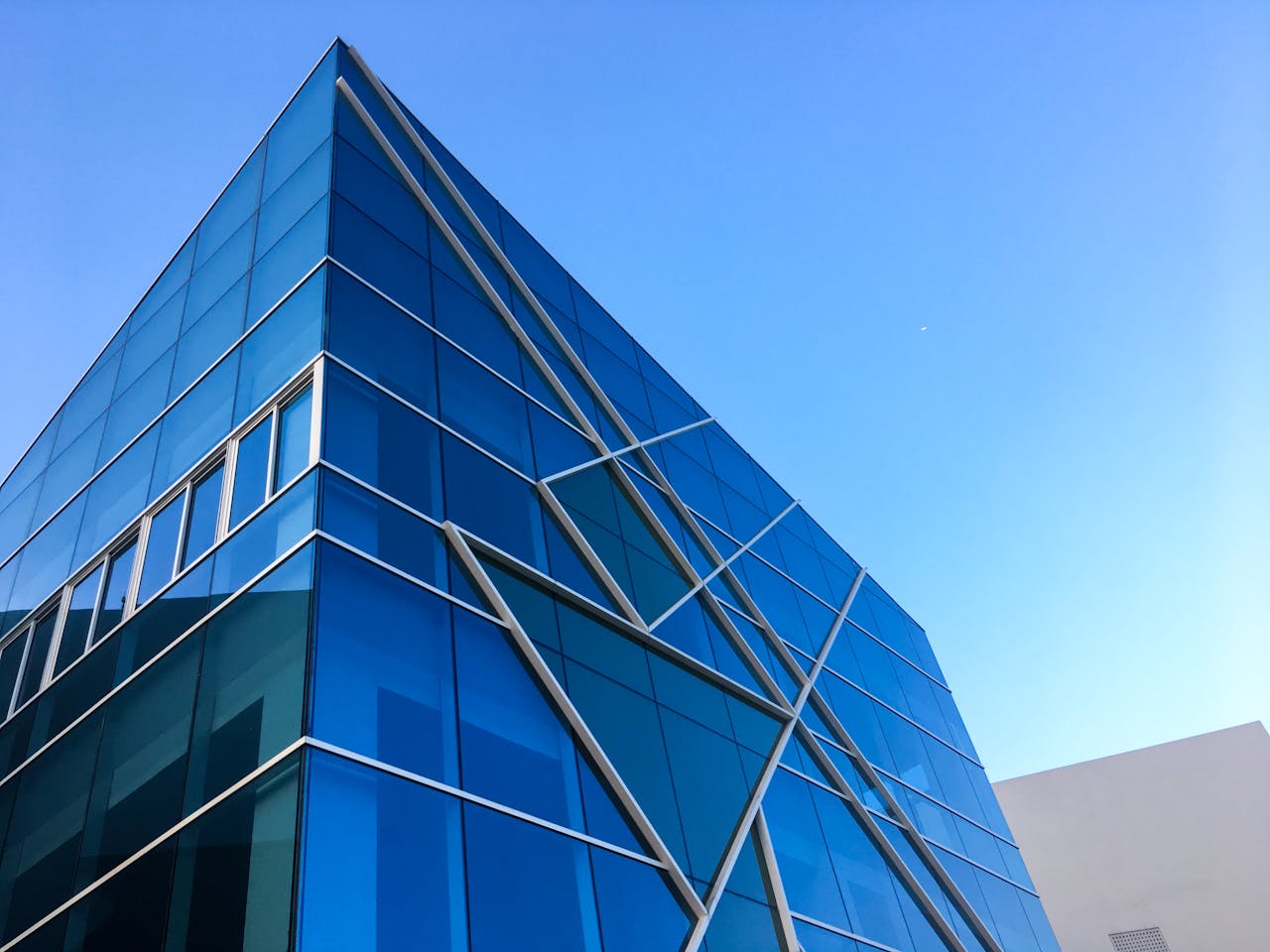
Interview and Discussion on the Potential of AI to Transform Healthcare with Dr. Ingrid Vasiliu-Feltes
Artificial intelligence (AI) plays a crucial role in the healthcare industry by helping doctors, patients and hospital administrators.
Setting the Scene for Artificial Intelligence & Healthcare
Artificial Intelligence (AI) is defined as computing systems which are capable of performing tasks that humans are very good at, for example recognising objects, recognising and making sense of speech, and decision making in a constrained environment. For the purposes of this article, Machine Learning and Deep Learning (Deep Neural Networks) are defined as sub-branches of AI. See the Appendix for a more detailed explanation of these areas.
Why Apply AI Towards The Healthcare Sector?
Healthcare systems were already under a substantial strain before the arrival of the Covid-19 pandemic. This strain has only increased since the pandemic and may cause challenges that persist for many years. It takes many years and costly resources to train healthcare workers. Specialist medical practitioners tend to be in short supply and often work long hours. Furthermore, the Covid-19 crisis forced healthcare systems to often operate a remote access basis in many cases during the peak of the pandemic.
Source for image above: Visualcapitalist.com Deloite
Source for image above: Visualcapitalist.com
The changing demographics across many OECD countries including the US, Japan and much of Europe entails dealing with complex diseases associated with an ageing population. On the other hand, the rapidly growing populations of non-OECD countries (for example Nigeria) leads to challenges with sufficient availability of trained medical staff to cater for the healthcare needs of such a fast-growing population.
AI has the potential to alleviate many of the strains across the healthcare system when applied to assisting healthcare workers and thereby improve patient outcomes. It can also help make sense of the deluge of data that is being generated from fitness trackers (wearable devices), vast amounts of research literature and Electronic Healthcare Records (EHRs).
Accenture forecast that AI clinical healthcare applications may generate savings of $150 billion per year by 2026 across the US healthcare sector.
Interview Session
Our interview discussion focused on the barriers to scaling AI and data science across the healthcare sector and how to resolve them. In particular the challenges around culture, data, and risk evaluation.
Imtiaz Adam
Thank you Dr. Ingrid Vasiliu-Feltes for giving your time.
A lot of discussions have occurred about the potential for AI in healthcare, and the potential to transform the sector. At a very high level, where do you see the potential benefits over the next three to five years, and also what barriers do you anticipate for achieving those?
As you possess a clinical background it would be fascinating to hear your thoughts on the potential of AI from a clinical perspective and the potential for AI in the era of personalized and precision medicine.
Dr. Ingrid Vasiliu-Feltes
Thank you for allowing me to share some of my insights. Definitely, from a healthcare and life sciences perspective, if we look into the next decade, I hope that some of the traditional silos will disappear so that we can adopt a more cohesive, comprehensive way of deploying AI, across multiple clinical disciplines, but also across the whole spectrum of disease from wellness, disease prevention, monitoring, diagnosis, chronic disease management, long-term rehabilitation, and even longevity.
I like to always emphasize that we've over-focused on certain niche domains such as oncology, however many other opportunities were missed that could contribute to optimizing global health. The other aspect that I think is important is to extrapolate the lessons learned from other industries and adapt them to healthcare and life sciences while safeguarding the privacy and security of confidential data.
However, we must not stifle innovation and aim to transform healthcare to impact global health and population health at scale. AI plays a key disruptive role and can change the paradigm in global health outcomes.
Imtiaz Adam
Thank you. That's an insightful overview. As you alluded to the challenge of validated data sets, what is your opinion about the potential for new techniques like federated learning and differential privacy to be adopted by key global stakeholders? Also, do you see much interest in the industry and across healthcare for collaborative efforts to achieve definitive real-world proof that these novel techniques can redefine the current model?
Dr. Ingrid Vasiliu-Feltes
Great question. Sadly, we had to experience the latest pandemic for some leaders to realize the value of collaboration, and changing some of the resistance. I'm a big believer in federated learning models and the creation of AI-enabled global data exchanges for the greater good. I think those could accelerate our progress and optimize outcomes.
The recently developed vaccines are great examples of how breaking silos and global cooperation led to a shorter R&D life cycle and many lives were saved.
On another note, legacy systems are witnessing a lot of pressure from new entrants into the market and the vibrant startup ecosystem. These are forcing traditional legacy healthcare systems to finally understand the need to rethink their data sharing strategy. Furthermore, some of the emerging technologies offer us now the opportunity to have optimized privacy and security systems in place. The convergence of AI and ledger technologies for example can offer dynamic informed consent, granular access control, and optimized confidentiality while exchanging crucial data vital for public health.
Hence, exponential technology adoption and embracing a novel mindset that promotes self-sovereignty will cause a paradigm shift in healthcare and life sciences. Consumers will be more empowered and able to share their data. Democratizing access to data and crowdsourcing data will be a trend that we'll see over the next few years while the global business ecosystem gets ready for web 3.0.
Imtiaz Adam
Can you perhaps elaborate on the quite interesting point that you made about COVID accelerating digital transformation in healthcare?
During the COVID pandemic, we have witnessed an acceleration of digital transformation and digital innovation. I draw the example of my own family, where four of my family members happen to be medical practitioners, three of them hospital medics, and the other one being a physician, a GP, had the challenge whereby during the initial COVID wave the medical practices were remote video only. Suddenly patients and medical practitioners had to adapt to a digital experience for medical services.
Going forwards there is the Metaverse anticipation with augmented reality, virtual reality, or mixed reality impacting healthcare delivery and patient engagement. Can you speak to the potential of combined technologies and their impact on personalized health?
Dr. Ingrid Vasiliu-Feltes
We currently notice a big divide in the ecosystem. On the one hand, we have the entrepreneurial community and the innovation labs or institutes that drive these trends. However, on the other hand, we have legacy organizations have a very hard time adjusting their risk models and their regulatory guidelines to accommodate for these transformative changes.
We also have to emphasize the exponential increase in the number and the average size of investments, as well the boundless creativity that breaks boundaries we could not imagine just a few years ago. By combining AI, AR XR, VR, blockchain, next-generation sequencing, bioimplants, remote sensors, and other types of emerging technologies we can disrupt healthcare. However, the challenge will be whether we are going to be able to adjust the regulatory and compliance guidelines, as well as relevant law at the same pace, as we are now experiencing a 5-10 years lag.
Imtiaz Adam
I think that's a great point, because often with healthcare, and you mentioned the regulatory, compliance, and legal system, as well, has also tended to be conservative (small c) with the need to demonstrate extensive real-world evidence which is very costly for the startup enterprise as this may take not only months but years to determine efficacy and safety.
And hence while emerging and cutting-edge technology such as AI may deliver exciting benefits to areas across healthcare, there remains an important role to play in protecting the patient, the end-user, etc, and ensuring that privacy standards are upheld.
However, at the same time, many hospital administrators, and the same key legal decision-makers and policymakers, are from a generation that doesn't understand digital, and therefore there's an additional resistance, a fear of advancing technology and change.
Do you believe it is going to take time to attain more transparency so that regulators become comfortable? Is it going to be a change with the younger generation taking more senior positions across healthcare and other key roles in the global healthcare ecosystem?
Dr. Ingrid Vasiliu-Feltes
That is a very important question. I believe we're going to see two drivers. One powerful driver is the new generations who are accustomed to living in a digital ecosystem. They expect to interact dynamically and immersively with the healthcare and life sciences, just like they do with other industries like entertainment, retail, or finance.
A second driver will be a broader demographic consumer-centricity that will cause a tipping point.
The entrepreneurship ecosystem is growing traction exponentially and at one point it will demand regulatory and legal responses to adjust to these large-scale adoption trends.
Telehealth is a great example. Take the situation before and the latest COVID pandemic. Before Covid there was still resistance, however now it's considered a required alternative. Genomics is another great example. A few years ago one genomic test was $100,000. And it was considered almost science fiction. Now we have hundreds of startups, just focusing on genomics, and some of them are already combining genomics, AI and blockchain, and other digital health components like remote sensors or biometrics. So total transformational change within just two-three years in the Genomic and Precision medicine space.
Imtiaz Adam
That's a great observation because if you think about it, technology is moving so fast, AI, Blockchain, computer vision, etc. that within two to three years, AI algorithms today will be surpassed by other algorithms that will be the cutting edge including areas of explainable AI and causal reasoning, and maybe the inertia that we see, resistance that we see better from the regulator from those people running hospitals may look at today's technology two to three years from and think you know, this is not so scary. We're now comfortable with this, being allowed, for example, whether it be text analytics or computer vision. In line with the point you made, which is that medicine, in theory, is about data and observation, isn't it?
And there's so much data sitting there in electronic health records and increased adoption of wearables and other fitness trackers generating data that it will arrive at the point you made earlier about being able to undertake predictive and preventative medicine, which hopefully could also reduce the strain on the overall system.
And if we're able to use text analytics, etc, to look in the electronic health records, and predict who might be at high risk, we might be able to relieve some of the stress on the system and gain optimal primary intelligence.
Dr. Ingrid Vasiliu-Feltes
Yes, absolutely. I concur with your thoughts on that topic. But I would also share that I believe in augmenting our digital ethics advocacy efforts to educate the consumers and C-suites on what AI means and reduce or eliminate some of the misconceptions. Because in all other industries, except healthcare and life sciences, C suite leaders are much more open to it and even racing to adopt it. Revising our messaging and clarifying that we aim to augment human intelligence would facilitate the adoption of AI in healthcare and life science. There are tremendous opportunities to impact global health through AI-enabled digital twins for healthcare and life sciences, in silico clinical trials, having synthetic datasets, and deploying AI for smart health cities.
Imtiaz Adam
Well, I'd be very fascinated with a digital twin of myself and able to see, objectively, what's going on with my digital twin.
It would take the personalized experience to a new dimension!
Moreover, you raised a point around longevity, and we've got this interesting dynamic in the world whereby some countries are facing an increasingly aging population and we must create solutions to address the quality of life for the aging population.
Another global challenge we are facing as a society is providing healthcare resources for a fast-growing population. And maybe, that opportunity to augment healthcare workers and medics, maybe most immediately applicable in the countries where the population is growing rapidly, there's often the challenge, generally speaking, that the healthcare resources are more limited due to economics and the time and cost that it takes to educate and train medics, their highly trained medics, and healthcare workers they'll often look to go to the US, Europe, Canada, Australia as examples for better paid healthcare jobs, which exacerbates the problem in locally non-OECD countries.
Hence maybe augmented strategic intelligence capabilities might be deployed more quickly in those regions because of the need, or China as they’ve invested so heavily into AI, big data, and 5G infrastructure.
I don't know if you have thoughts on that, or if that might be how it ends up playing out in the future with the emerging markets moving faster than the developed world with AI in healthcare?
Dr. Ingrid Vasiliu-Feltes
Absolutely. And we have the same example for telehealth again. In countries where you have no access to or poor quality healthcare, you will be very happy to have a telehealth option that allows you access to the most appropriate specialty care in whatever city you want or at a time convenient to you.
In emerging markets, because the choices are very different, and it's a necessity for survival, you'll have a higher adoption very quickly. For more developed countries with complex healthcare systems, we must deploy novel and different business models. The aim is not to replace in-person care; telehealth and virtual care are changing the paradigm and can ease the workload for physicians, nurses, and any other allied health practitioners.
Imtiaz Adam
That's a very good point. And this is the issue of regulators and those running our healthcare systems in general, becoming more comfortable with AI, in general, and technology in general. When we think of the explosion in AI, deep neural networks, etc, machine learning over the last decade or so, a lot of it is driven by this explosion in both the internet, mobile, smart mobile, and social media.
And now as we bring AI into the real world in some way, whether it be autonomous driving, whether it be healthcare, or other sectors, which touches people's lives. But apart from the data privacy issue here, a big challenge that's been mentioned a lot is explainability. And understanding why. So why did the AI or the deep neural network make that decision? And of course, one could argue five, six years ago, that wasn't so important in AI research, because the tech majors and researchers were focused on recommendation and other marketing algorithms for social media and eCommerce whereby if the algorithm recommends the wrong fashion item to the wrong movie, nobody dies, there's no injury, but with healthcare or an autonomous vehicle that can have real-life consequences, somebody can get seriously injured False positives, false negatives, etc. And hence being able to understand why they made that decision.
Of course, there have been a lot of advancements in the last year or two on improving explainability even with deep neural networks. So whilst we're perhaps not fully there yet, we are getting much better than where we have been before and there is exciting and promising research and development emerging ranging from Transformer models with a self-attention mechanism that is more interpretable, to hybrid Neurosymbolic AI, Liquid Neural Networks, and Concept Whitening for Convolutional Neural Networks. Do you believe that this is one of the areas that the regulators have been pushing back on? Do you believe that by improving AI explainability it will be easier for the regulators to get comfortable?
Dr. Ingrid Vasiliu-Feltes
I have a split view on that topic. On the one hand, my answer is a decisive yes. I'm a major advocate for AI explainability. However, we need a balance between how much explainability is needed, when it can hinder progress or performance, and how much is required for consumers and regulators to trust AI products or services.
Imtiaz Adam
I certainly agree with you on the point that if the explainability of models requires a Ph.D. in Data Science to sit down in a hospital and undertake a complex exercise in interpreting a model, then that may be a costly exercise in terms of computation and human resources. However, if we're talking about systems like the liquid neural networks, an AI that was demonstrated with an autonomous vehicle was sparse networks, we obtain causal reasoning and explainability, which could be a future direction for AI R&D in healthcare.
Whilst that hasn't been applied to healthcare yet, perhaps such approaches may get there in two to three years. And I recall there was recent research by MIT with a novel model called MILAN that automatically describes, in natural language, what the individual components of a neural network do. Therefore, AI R&D is improving on model interpretability, which hopefully, can make regulators much more comfortable perhaps within the next couple of years.
Dr. Ingrid Vasiliu-Feltes
There are examples where we can showcase high explainability, however, data sources were biased or not validated. I'm trying to also caution that the whole process needs to be transparent and not just the algorithm
Imtiaz Adam
The data validity is key because otherwise, you get garbage in garbage out, right, as it doesn't matter if you got the best algorithm in the world. It's just not going to be effective at all. So this issue about data comes back to the data, data science, machine learning, etc, which is, as you say, making sure your data you've got good clean data, or that effort is put in the data side. And often, I do concur that a lot of the work does have to be on the data side, before you ever get to the algorithm, and make sure you look at it carefully so that you're transparent with the data. There's heterogeneity in there. Thus, it's not just one racial group or other scenarios where heterogeneity is not sufficient.
Dr. Ingrid Vasiliu-Feltes
I think that's crucial, because honestly, every time I've seen a data breach or I had an opportunity to evaluate digital ethics risk, it has always been related to process and human interactions. Many times they are related to poor interoperability planning and faulty human-computer interactions, or suboptimal data governance.
I think having synthetic datasets might be a viable solution to protect us from ethical violations. Furthermore, having crowdsourcing might be feasible as well for specific situations and use cases.
Appendix Resource Guide for AI & Healthcare
Artificial Intelligence (AI) deals with the area of developing computing systems which are capable of performing tasks that humans are very good at, for example recognising objects, recognising and making sense of speech, and decision making in a constrained environment.
Machine Learning is defined as the field of AI that applies statistical methods to enable computer systems to learn from the data towards an end goal. The term was introduced by Arthur Samuel in 1959.
Neural Networks: are biologically inspired networks that extract abstract features from the data in a hierarchical fashion. Deep Learning refers to the field of Neural Networks with several hidden layers. Such a Neural Network is often referred to as a Deep Neural Network. Many of the advancements relating to AI in healthcare have entailed applications of Deep Learning in relation to natural language applied to text analytics, medical imaging and also across drug discovery.
Machine Learning techniques may entail:
Supervised Learning: a learning algorithm that works with data that is labelled (annotated). For example learning to classify fruits with labelled images of fruits as apple, orange, lemon, etc.
Unsupervised Learning: is a learning algorithm to discover patterns hidden in data that is not labelled (annotated). An example is segmenting customers into different clusters.
Semi-Supervised Learning: is a learning algorithm when only a small fraction of the data is labelled.
Self-Supervised Learning: may be considered as a type of unsupervised learning as it meets the criteria of no labels being provided. “However, instead of finding high-level patterns for clustering, Self-Supervised Learning attempts to still solve tasks that are traditionally targeted by supervised learning (e.g., image classification) without any labelling available.” (Ta-Ying Cheng)
Machine Learning Applications may include:
Classification summarised by Jason Brownlee "is about predicting a label and regression is about predicting a quantity. Classification is the task of predicting a discrete class label. Classification predictions can be evaluated using accuracy, whereas regression predictions cannot."
Regression predictive modelling is summarised by Jason Brownlee "is the task of approximating a mapping function (f) from input variables (X) to a continuous output variable (y). Regression is the task of predicting a continuous quantity. Regression predictions can be evaluated using root mean squared error, whereas classification predictions cannot."
Clustering summarised by Surya Priy "is the task of dividing the population or data points into a number of groups such that data points in the same groups are more similar to other data points in the same group and dissimilar to the data points in other groups. It is basically a collection of objects on the basis of similarity and dissimilarity between them."
Applications of AI in Healthcare
Medical Imaging: Deep Neural Networks have vast potential to assist in the process of classifying images for example whether an image shows signs of cancer. Recently an imaging tool that reads chest X-rays without the involvement of a radiologist was approved by the EU.
Natural Language: analytics across EHRs, mining research journals, speech to text (and vice versa) interactions;
Drug Discovery: Applications of AI technology towards developing novel therapeutics drugs has potential to significantly reduce time to develop drugs to treat complex diseases as well as costs. Examples of applying AI for DeNovo drug discovery include Insilico Medicine whose AI-discovered and AI-designed IPF drug recently entered Phase 1 trials.
Robotic Surgery: AI has potential to assist surgery in both preoperative and intraoperative planning along with surgical robotic use cases. Collaborative robots with AI may be applied with computer vision towards the analysis of scans to detect cancerous cases. Unexpected or missing steps in real-time may be identified when analyzing videos of laparoscopic video surgery.
Clinical Trialing: the application of AI technology may assist in delivering enhancements in clinical trialing from patient recruitment to adherence monitoring and data collection disrupting the process and enabling new therapies to reach the market more quickly. Furthermore, AI may be applied for real-time analytics of data generated from wearable devices for monitoring during clinical trials.
Source for image above: PLOS Medicine
When applied alongside standalone 5G networks, AI may also improve the delivery of remote medicine including real-time analytics.
The era of preventative medicine may arrive
Barriers Towards AI in Healthcare
Data and privacy: Machine Learning and in particular Deep Neural Networks with Supervised Learning require large amounts of quality labelled data to learn. Furthermore, the data needs quality annotation (labelling) for the ground truth otherwise the model will learn garbage and give out garbage.
In addition, it is important to ensure heterogeneity in the source data if we are developing clinical applications to be applied across the wider population rather than rely upon data generated across one specific group. Hence rigorous testing and standards across data sources are essential foundations for applications of Data Science across healthcare. The “S” and “G” parts of ESG relating to Social and Governance become key for scaling AI across healthcare due to the complex issues of ethics, good data standards and rigorous testing both before and after clinical development.
However, much of the data across healthcare is siloed in disparate servers across different healthcare facilities. Moreover, patient privacy regulations limit access to the data for example with HIPAA in the US and GDPR in Europe.
Over time, it is anticipated that anonymisation techniques, greater quality synthetic data and in particular advances in Federated Learning with Differential Privacy may help alleviate this challenge.
Explainability: model interpretability has been viewed as a barrier to scaling techniques such as Deep Learning across healthcare. Often the healthcare practitioners and indeed the end user patient will wish to know the “why” for a reason that a Machine Learning model is classifying a particular example as “X” instead of “Y”.
In recent time advances have been made with explainable AI due to the following factors:
-
Transformers with self-attention mechanisms are more interpretable relative to certain other Deep Learning models such as Convolutional Neural Networks. Moreover, code may be applied to Transformers to further add model interpretability;
-
Concept Whitening has been applied to Convolutional Neural Networks to gain greater model interpretability;
-
Emerging techniques such as Neural Circuit Policies with Liquid Neural Networks provide model explainability;
-
Hybrid AI techniques such as Neurosymbolic AI that combine Deep Neural Networks with Symbolic AI have been demonstrated to enhance model interpretability;
-
Recent Research from MIT entitled MILAN proposed a new method automatically describes, in natural language, what the individual components of a neural network do.
It is anticipated that the above techniques may translate into greater model interpretability across AI in healthcare in the next two to three years.
Furthermore, the field of research and development causal reasoning and AI is also developing and it is anticipated that causal inference in AI and healthcare will also progress over the next few years.
Source for image above: Artificial Intelligence in Healthcare needs causality
Techniques such as Neural Circuit Policies with Liquid Neural Networks and Neurosymbolic AI approaches are expected to gain momentum over the next few years in real-world applications including potential for machine reasoning within healthcare applications.
About the Authors
Dr. Ingrid Vasiliu-Feltes is an alumnus of Columbia University Vagelos College of Physicians and Surgeons with a residency & fellowship and also obtained an Executive MBA in healthcare management & policy. Thereafter Dr. Ingrid Vasiliu-Feltes is ranked as a leading global influencer in Health Tech and is very prominent in the startup and angel investment sectors. Furthermore, Dr. Ingrid Vasiliu-Feltes has also been active in thought leadership around the potential of Web 3.0 and the potential for the Metaverse.
Imtiaz Adam is ranked as a leading thought leader in AI and holds a postgraduate degree in Computer Science with research in AI.
Trending
-
1 How Does SaaS Differ From IaaS And PaaS?
Fabrice Beaux -
2 Single Page Applications vs Multi-Page Applications
Fabrice Beaux -
3 Top 7 Effective Strategies for Multi-Language Website Development
Fabrice Beaux -
4 Boost Engagement to Infinity and Beyond: Unleashing AI-Driven Support
Anas Bouargane -
5 The Cheapest And Most Beautiful Stickers in CS2
Daniel Hall
Comments