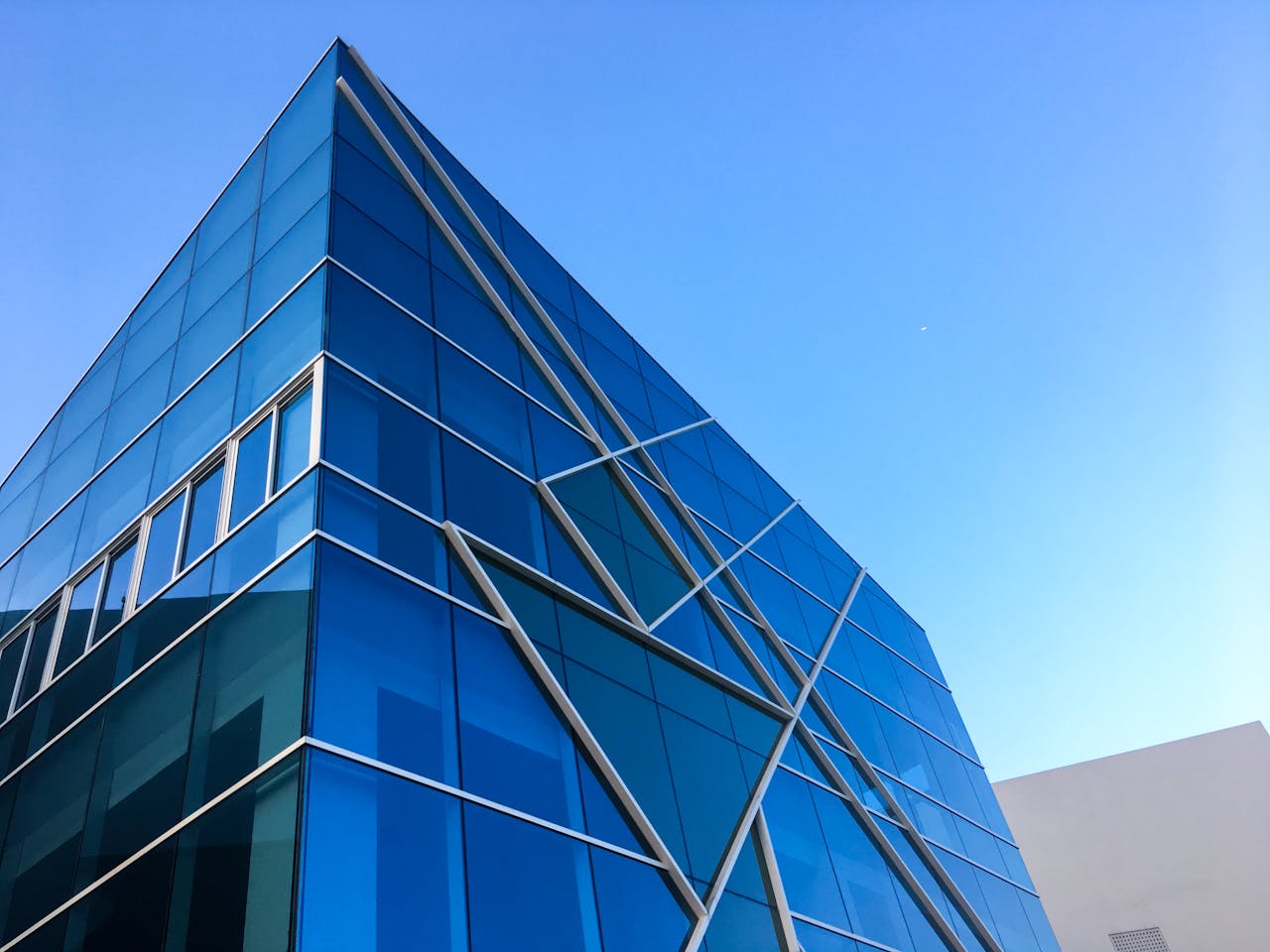
The State of Artificial Intelligence
Artificial intelligence (AI) is stepping up in more concrete ways in blockchain, education, internet of things, quantum computing, arm race and vaccine development.
AI-first approaches have taken biology by storm with faster simulations of humans’ cellular machinery (proteins and RNA). This has the potential to transform drug discovery and healthcare.
Transformers have emerged as a general purpose architecture for machine learning, beating the state of the art in many domains including natural language planning (NLP), computer vision, and even protein structure prediction.
AI is now an actual arms race rather than a figurative one.
Internet vs. Artificial Intelligence Era
Organizations must learn from the mistakes we made with the internet, and prepare for the future of AI.
DHL and FedEx are centuries old logistics companies that have successfully made the jump into the internet age. Conversely, Eastman Kodak and Polaroid were camera companies that could not successfully make the jump into the digital age. The next twenty years will show which companies will make the jump successfully from digital to AI.
I can't stress the point of Strategic Data Acquisition enough. Strategic Data Acquisition. I'll write it again. Strategic Data Acquisition. Almost any reputable Data Science Team can get their hands on some great computing power via Nvidia, AWS, GCP or Azure. Papers are widely published on various approaches to develop the deepest and widest neural network, but the one thing that these AI companies have done to build a moat around themselves is capturing their users' data.
Supervised Learning, Unsupervised Learning and Beyond!
The next point that struck a chord with me was the increasing level of sophistication in AI approaches while I'll outline below.
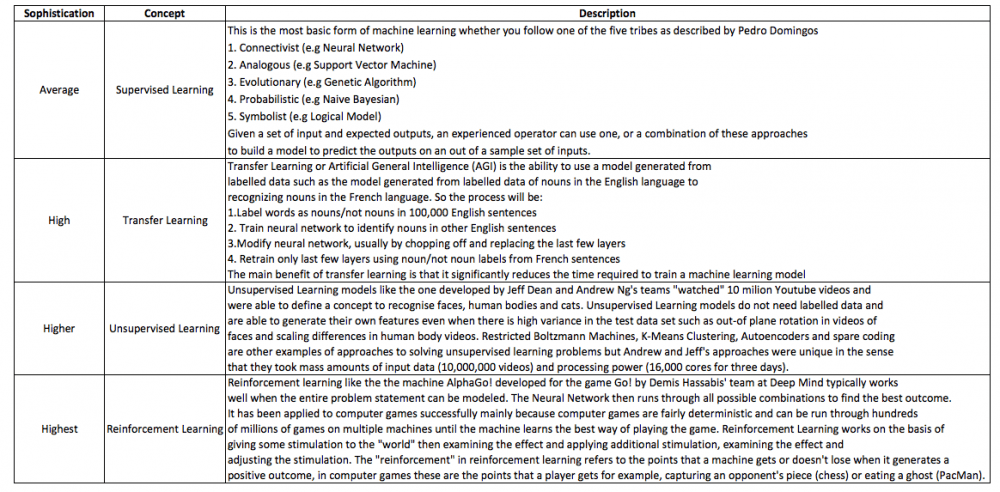
Below is an image that outlines, in more detail the Representational, Evaluation, and Optimizational levels of the Five Tribes of ML as described by Pedro Domingos.
Building an AI Centre of Excellence
This year, we have seen AI become increasingly pivotal to breakthroughs in everything from drug discovery to mission critical infrastructure like electricity grids.
With lessons learned from operationalizing AI, the emphasis is shifting from shiny new models to perhaps more mundane, but practical aspects such as data quality and data pipeline management.
With the increasing power and availability of machine learning models, gains from model improvements have become marginal.
As machine learning is increasingly used in real-world applications, the need for a solid understanding of distributional shifts becomes paramount. This begins with designing challenging benchmarks.
Trending
-
1 How Does SaaS Differ From IaaS And PaaS?
Fabrice Beaux -
2 Single Page Applications vs Multi-Page Applications
Fabrice Beaux -
3 Top 7 Effective Strategies for Multi-Language Website Development
Fabrice Beaux -
4 Boost Engagement to Infinity and Beyond: Unleashing AI-Driven Support
Anas Bouargane -
5 The Cheapest And Most Beautiful Stickers in CS2
Daniel Hall
Comments