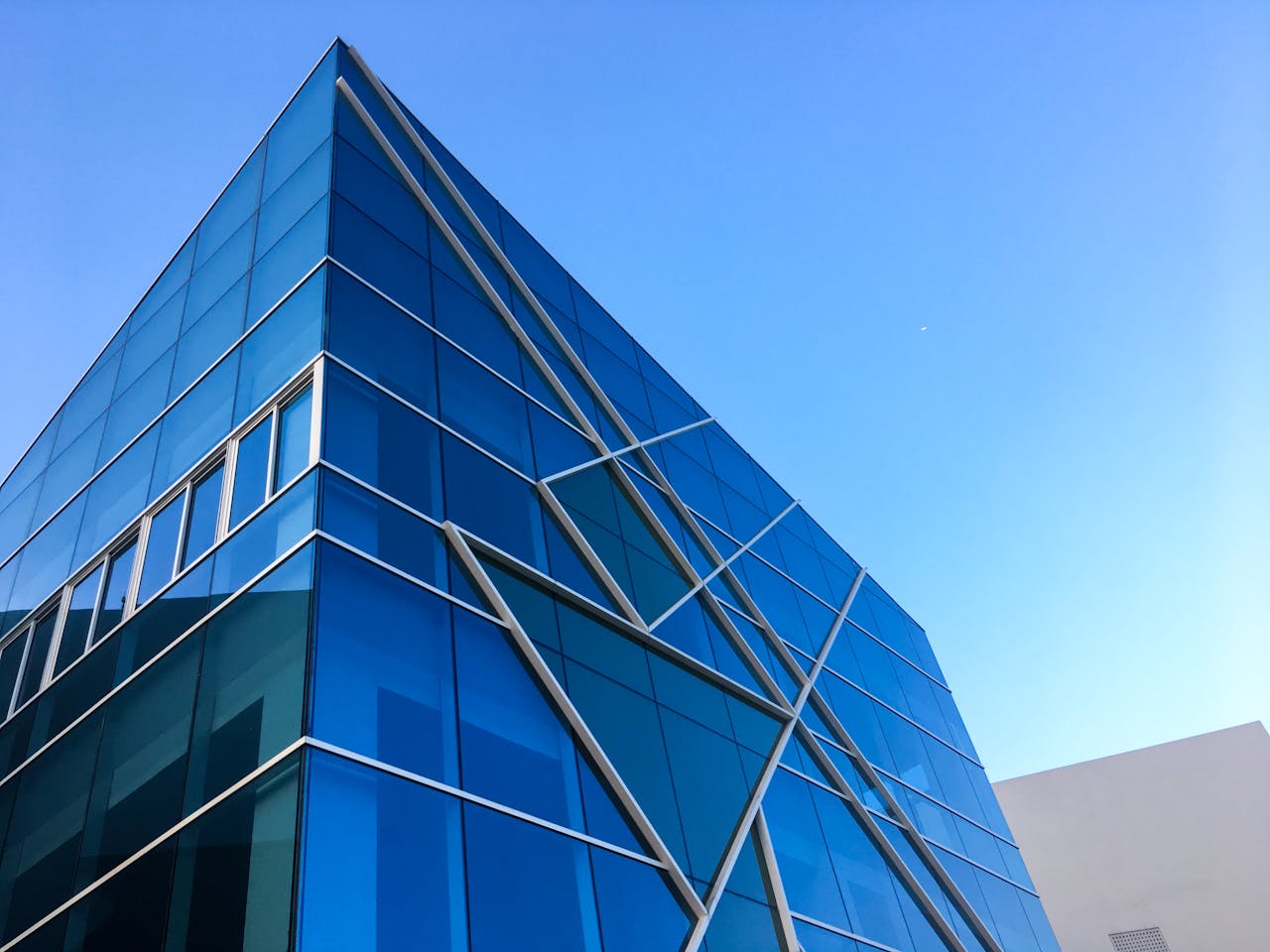
Understanding the Challenges and Progress in AI Alignment
Artificial Intelligence (AI) has become an integral part of our daily lives, influencing everything from how we communicate to how we make decisions.
As AI systems continue to evolve and advance, the need for aligning their goals with human values and intentions becomes increasingly critical. We will delve into the complex landscape of AI alignment, exploring its challenges, current research trends, and the potential impact on society.
I. Exploring AI Alignment
AI alignment refers to the process of ensuring that artificial intelligence systems act in accordance with human values and goals. The crux of the issue lies in the potential misalignment between the objectives of AI systems and the values held by humans. Left unaddressed, misalignment could lead to unintended consequences, posing risks to both individuals and society at large.
- The Alignment Problem
The alignment problem encapsulates the challenge of creating AI systems that understand and adhere to human values. As AI systems become more sophisticated, their behavior may deviate from human expectations, raising concerns about the ethical implications of their actions. Addressing the alignment problem is crucial to harnessing the benefits of AI while minimizing the associated risks.
- Types of AI Alignment
There are several approaches to AI alignment, each with its own set of challenges and considerations:
a. Goal Alignment: Focusing on aligning the objectives of AI systems with human values, ensuring that the AI pursues goals that are beneficial and ethical.
b. Value Alignment: Emphasizing alignment at a deeper level, seeking to imbue AI systems with a fundamental understanding of human values and ethical principles.
c. Robustness Alignment: Ensuring that AI systems remain aligned even in the face of unforeseen circumstances or adversarial attempts to manipulate their behavior.
II. Challenges in AI Alignment
The journey towards achieving AI alignment is rife with challenges, reflecting the intricate nature of aligning artificial intelligence with human values. Several key challenges include:
- Ambiguity in Human Values
Human values are complex, multifaceted, and often subjective. Aligning AI with these values requires a nuanced understanding of cultural, ethical, and individual variations, posing a significant challenge for developers and researchers.
- Value Drift
The concept of value drift refers to the potential divergence of AI systems from their intended alignment over time. As AI adapts and learns from its environment, it may unintentionally deviate from the desired alignment, necessitating continuous monitoring and adjustments.
- Scalability
Scalability is a critical challenge in AI alignment, especially as systems become more powerful and widespread. Ensuring alignment at scale involves developing frameworks that can accommodate a diverse range of applications and contexts.
- Adversarial Manipulation
AI systems may be vulnerable to adversarial manipulation, where external actors deliberately attempt to influence the system's behavior for malicious purposes. Building AI systems that are robust against such manipulation is a crucial aspect of alignment.
III. Approaches to AI Alignment
Researchers and practitioners employ various approaches to tackle the challenges of AI alignment. These approaches range from theoretical frameworks to practical methodologies, each contributing to the ongoing discourse on aligning AI with human values.
- Value Learning
Value learning involves teaching AI systems to understand and adopt human values. This approach aims to imbue AI with a comprehensive understanding of ethical principles, enabling it to make decisions that align with human preferences.
- Inverse Reinforcement Learning
Inverse reinforcement learning seeks to infer the underlying values or preferences of humans by observing their behavior. By understanding human actions and decisions, AI systems can better align their objectives with the implicit values of individuals.
- Cooperative Inverse Reinforcement Learning
Cooperative inverse reinforcement learning extends the concept of inverse reinforcement learning by incorporating feedback and collaboration between AI systems and humans. This iterative process allows for ongoing refinement of alignment based on real-world experiences.
- Formal Verification
Formal verification involves mathematically proving that an AI system adheres to a specified set of rules or values. This approach aims to provide rigorous guarantees of alignment, offering a level of assurance that the system will behave as intended in all circumstances.
IV. Progress in AI Alignment
As the field of AI alignment continues to mature, notable progress has been made in addressing its challenges. From theoretical advancements to practical implementations, researchers are actively contributing to the development of alignment solutions.
- Research Initiatives
Leading research institutions, such as OpenAI, DeepMind, and the Future of Humanity Institute, have dedicated efforts to understanding and mitigating the risks associated with AI alignment. These initiatives focus on advancing the theoretical foundations of alignment and developing practical tools for implementation.
- Collaborative Efforts
The complexity of AI alignment necessitates collaboration between researchers, developers, policymakers, and ethicists. Collaborative efforts aim to foster a multidisciplinary approach, drawing on diverse expertise to tackle the multifaceted challenges of alignment.
- Ethical Guidelines
The development of ethical guidelines for AI is gaining traction as a means to ensure alignment with human values. Organizations and industry bodies are working to establish principles that prioritize transparency, fairness, and accountability in AI systems.
- Public Awareness and Engagement
Raising public awareness about the challenges and implications of AI alignment is crucial for fostering a collective understanding of the issues at stake. Engaging the public in discussions about the ethical use of AI helps ensure that diverse perspectives are considered in the alignment process.
V. Future Directions and Considerations
The future of AI alignment holds both promise and uncertainty. As the field evolves, several key considerations and directions will shape the trajectory of alignment research and implementation.
- Continued Research and Innovation
Ongoing research and innovation are essential for advancing the field of AI alignment. Embracing a forward-looking mindset, researchers will explore novel approaches, refine existing methodologies, and address emerging challenges to ensure the continued progress of alignment efforts.
- Ethical Governance
Establishing robust ethical governance frameworks is imperative for guiding the responsible development and deployment of AI systems. Policymakers and industry stakeholders must collaborate to create standards that prioritize alignment, fairness, and accountability.
- Human-AI Collaboration
The concept of human-AI collaboration emphasizes the symbiotic relationship between humans and AI systems. Fostering collaboration allows for the collective harnessing of human expertise and AI capabilities, ensuring alignment with human values in decision-making processes.
- Education and Awareness
Educating both professionals and the general public about AI alignment is crucial for building a knowledgeable and engaged community. Workshops, educational programs, and public discourse will contribute to a broader understanding of the challenges and opportunities associated with aligning AI with human values.
AI alignment stands at the intersection of technological innovation, ethics, and societal impact. As we navigate the complex terrain of aligning artificial intelligence with human values, it is essential to approach the challenge with diligence, collaboration, and a commitment to responsible development. By addressing the multifaceted aspects of AI alignment, we can build a future where AI systems contribute positively to society while respecting the values and preferences of humanity.
Trending
-
1 How Does SaaS Differ From IaaS And PaaS?
Fabrice Beaux -
2 Single Page Applications vs Multi-Page Applications
Fabrice Beaux -
3 Top 7 Effective Strategies for Multi-Language Website Development
Fabrice Beaux -
4 Boost Engagement to Infinity and Beyond: Unleashing AI-Driven Support
Anas Bouargane -
5 The Cheapest And Most Beautiful Stickers in CS2
Daniel Hall
Comments