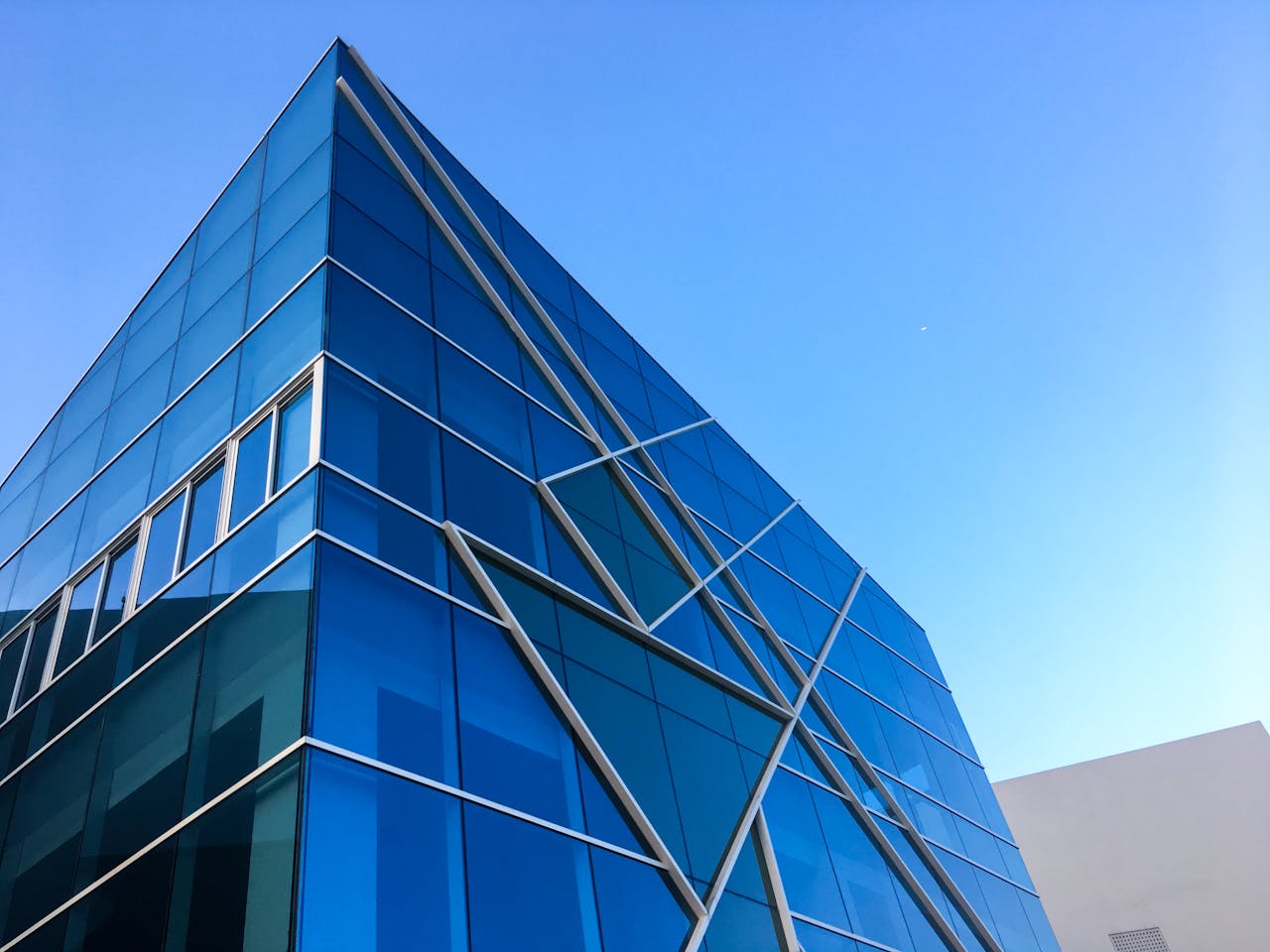
Unique Ways to Use AI in Data Analytics
Artificial intelligence is rapidly gaining popularity in a variety of industries — from healthcare and e-commerce to banking, manufacturing, and engineering.
But how can it actually help out in data analytics? For many, the actual application of Generative AI inside the data analytics ecosystem is unclear and vague. Let us shed some light on this topic and discuss the key use cases where businesses have used AI in their data analytics strategy to increase the value they get from their data. So keep reading and be inspired.
Use Case #1: Code Generation
A key use case for modern AI powered data analytics solutions is the practical implementation of large language models (LLM) in code development, which significantly speeds up the total development lifecycle. We do not consider Generative AI to be a complete replacement for well-structured code. However, the automatic generation of template code — or living repositories of previously written code for particular use cases — accelerates the speed of delivery teams when executed correctly.
Besides that, text-based LLMs can transform old code bases into target code bases and serve as a migration accelerator. A simple example of this is an analytics migration situation, such as moving Qlik Sense reporting right to Power BI. One of the essential elements of this project would involve transforming the proprietary Qlik syntax right into DAX code on the front end of the reporting. Typically, this would involve someone familiar with both tool bases. AI allows you to automatically convert basic expressions from Qlik syntax to DAX and deliver the solution more efficiently.
Use Case #2: Chat Bots and Virtual Agents
For those thinking about implementing a chatbot to their website, the arrival of broad-based LLMs has made the creation and deployment of chatbot systems easier than previously. Integrating chatbot capability into front-end analytics may provide depth and insight into current reports, in addition to visualizing them.
Cloud platforms are rapidly developing “data-in” capabilities that allow the implementation of cognitive search services and off-the-shelf LLMs against your own dataset, decreasing the barrier to entry. These chatbots may be smoothly incorporated into processes via API endpoints or native app deployment. Open-source frameworks may be installed in the same manner.
Use Case #3: Data-Driven Marketing
AI changes data analytics in marketing by allowing for targeted advertising, consumer segmentation, and customized suggestions. Machine learning algorithms examine customer behavior, demographic data, and purchase trends to determine target audience groupings and adjust marketing campaigns appropriately.
AI-driven recommendation engines, such as those used by Amazon and Netflix, use data analytics to provide customized recommendations for items, services, or content based on individual interests and previous interactions. Furthermore, sentiment analysis tools use social media data to evaluate public opinion, predict new trends, and analyze brand sentiment, allowing marketers to change their strategy in real-time for optimal effect.
Use Case #4: Apps, Automation, and Workflows
To create simple action/trigger response applications, lightweight application interfaces may make API calls to GPT and LLM models. Consider how GPT may be taught to emulate a customer service agent or to search a company's internal network for essential data and display it via a form-based interface. Basic automation may also be developed. As a result, you may automate email answers to incoming questions or emails that trigger an automated prompt.
Caveats for Generative AI
While Generative AI looks quite promising when it comes to data analytics, there are several concerns to consider before making it a part of your data strategy:
-
Lack of evidence;
-
Data security, intellectual property, and personally identifiable information risks;
-
Data accuracy;
-
Cost.
Although generative AI provides a lot of opportunities for businesses to grow and work with the data better, there are still some challenges and pitfalls to be aware of. If you employ the right approach, you can easily mitigate the risks and benefit from using artificial intelligence.
What to Expect?
Despite the issues that are harming the progress of AI, the new technology is still developing. Artificial intelligence can be used in a variety of industries and for different purposes. Data analytics is not an exception here. AI can come in handy when it comes to running data-driven marketing campaigns, process automation, and code generation. All these will for sure have a great impact on your business and revenue. So you should definitely make gen AI a part of your data analytics strategy.
Trending
-
1 How Does SaaS Differ From IaaS And PaaS?
Fabrice Beaux -
2 Single Page Applications vs Multi-Page Applications
Fabrice Beaux -
3 Top 7 Effective Strategies for Multi-Language Website Development
Fabrice Beaux -
4 Boost Engagement to Infinity and Beyond: Unleashing AI-Driven Support
Anas Bouargane -
5 The Cheapest And Most Beautiful Stickers in CS2
Daniel Hall
Comments